☆
1
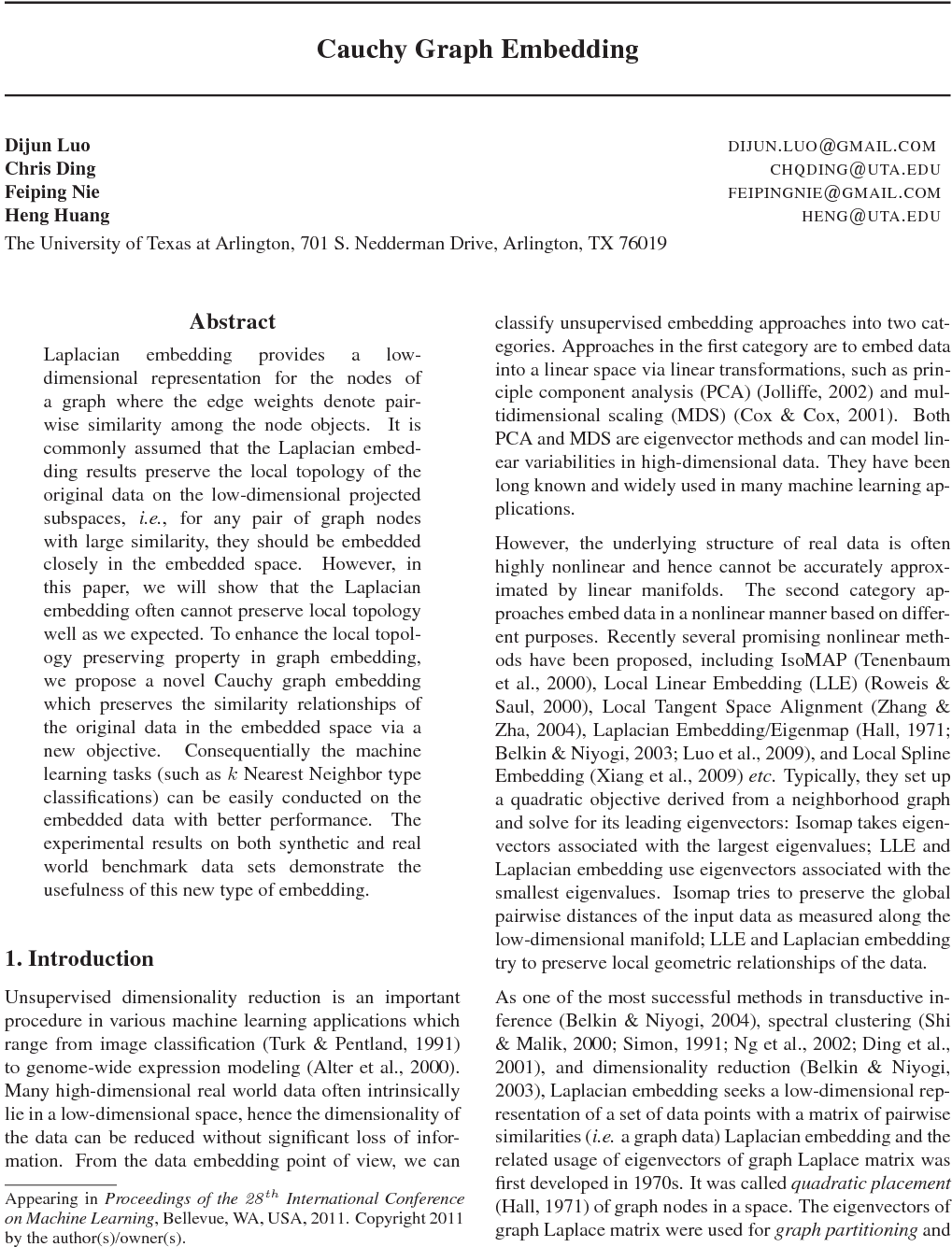
Authors:
Dijun Luo,
Chris Ding,
Feiping Nie,
Heng Huang
Liked by: Maximimi
Domains: Machine Learning
Tags: embedding
Liked by: Maximimi
Domains: Machine Learning
Tags: embedding
Uploaded by:
Stranger
Upload date: 2018-05-21 20:13:21
Upload date: 2018-05-21 20:13:21
Comments:
Edited by Maximimi at 2018-05-23 10:53:12
Edited by Sergey Kirgizov at 2018-05-30 10:31:57