☆
0
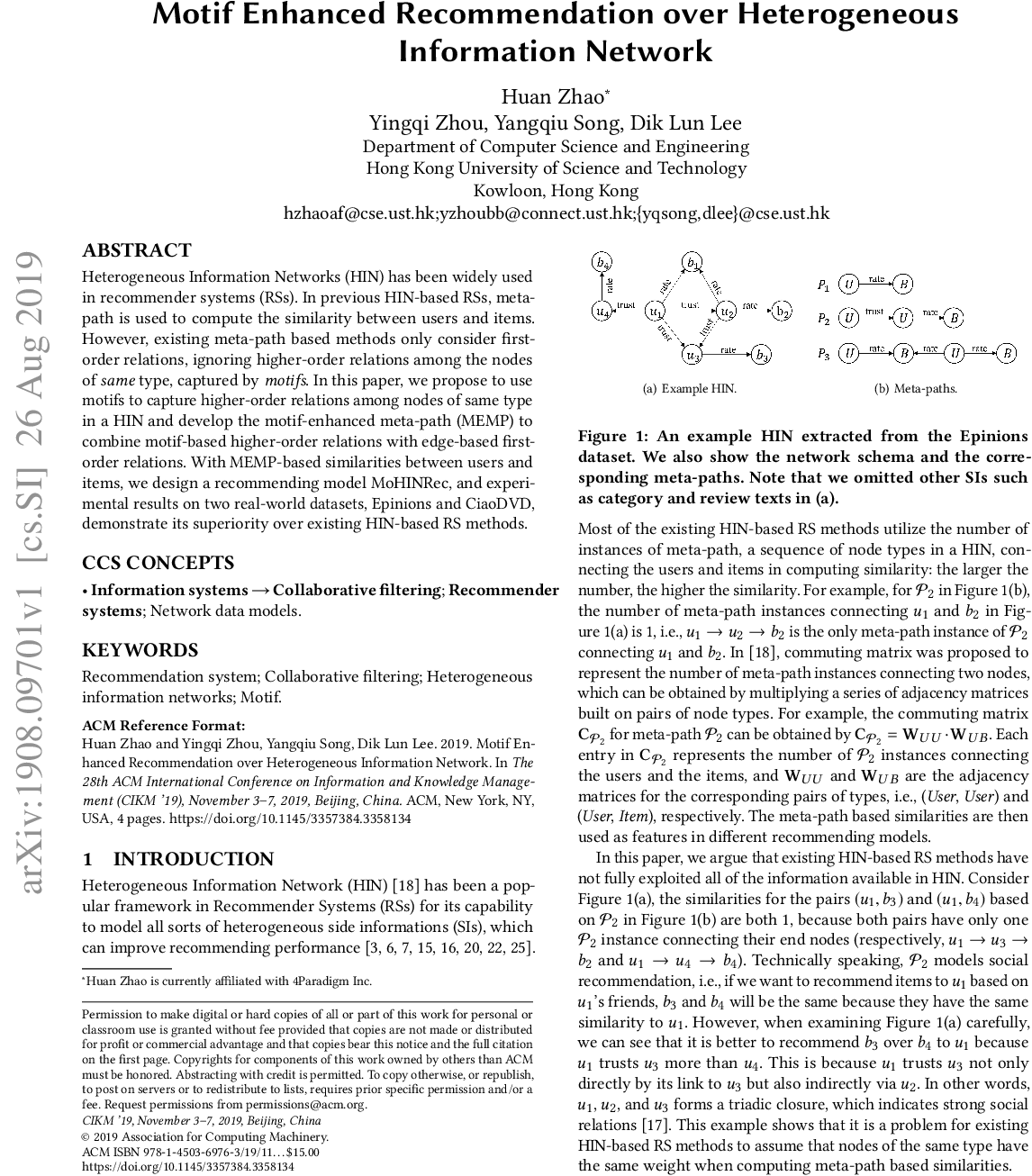
Authors:
Huan Zhao,
Yingqi Zhou,
Yangqiu Song,
Dik Lun Lee
Liked by:
Domains: Machine Learning, Recommendation
Tags: diversity, heterogeneous information networks
Liked by:
Domains: Machine Learning, Recommendation
Tags: diversity, heterogeneous information networks
Uploaded by:
Alt-Tab
Upload date: 2019-10-11 13:39:11
Upload date: 2019-10-11 13:39:11
Comments: