☆
0
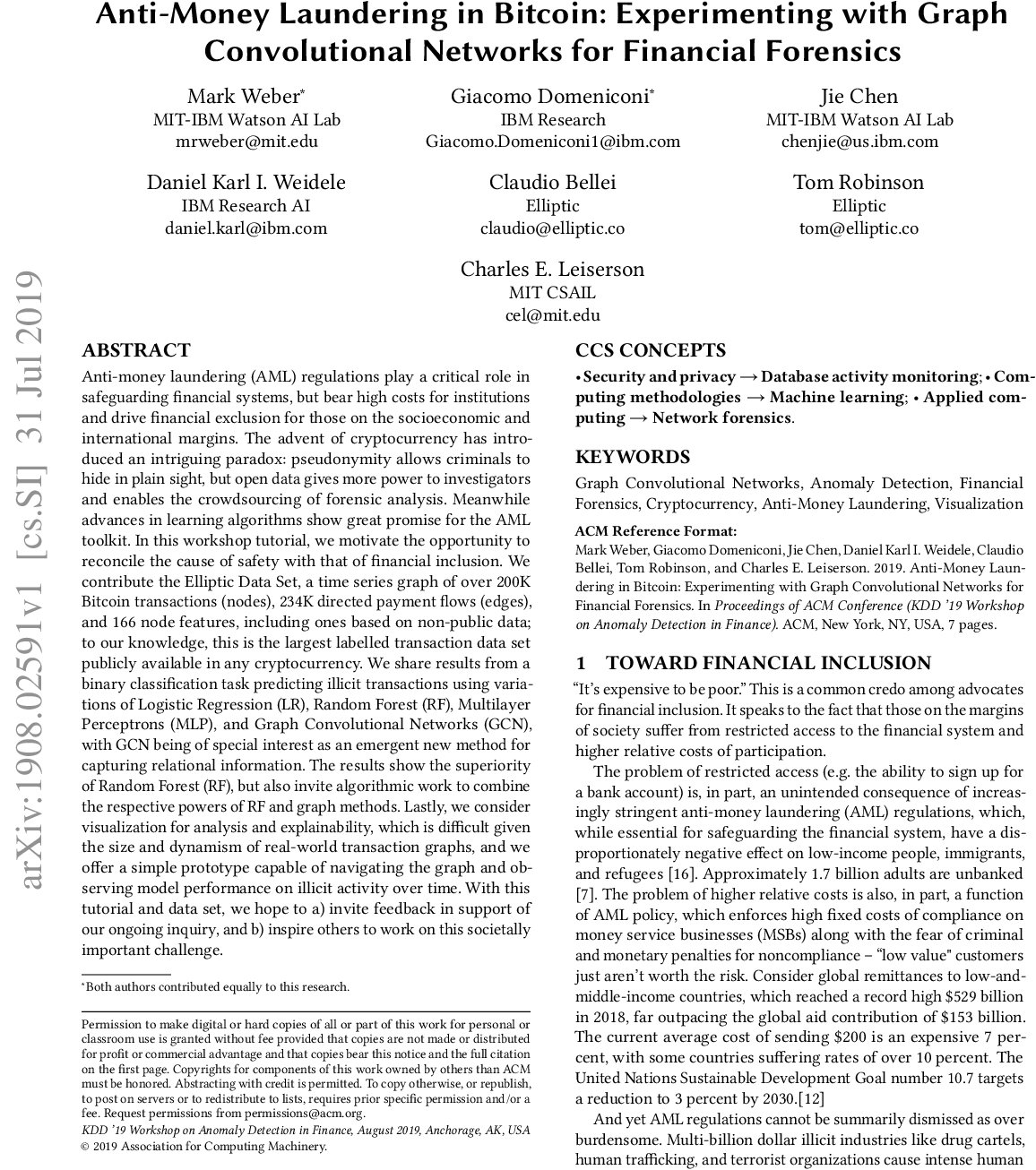
Authors:
Mark Weber,
Giacomo Domeniconi,
Jie Chen,
Daniel Karl I. Weidele,
Claudio Bellei,
Tom Robinson,
Charles E. Leiserson
Liked by:
Domains: Graph mining, Cyber security, Machine Learning, Data Analysis
Tags: bitcoins, learning, detection, aml, KDD2019
Liked by:
Domains: Graph mining, Cyber security, Machine Learning, Data Analysis
Tags: bitcoins, learning, detection, aml, KDD2019
Uploaded by:
Zorro
Upload date: 2019-09-08 11:46:43
Upload date: 2019-09-08 11:46:43
Comments: