☆
0
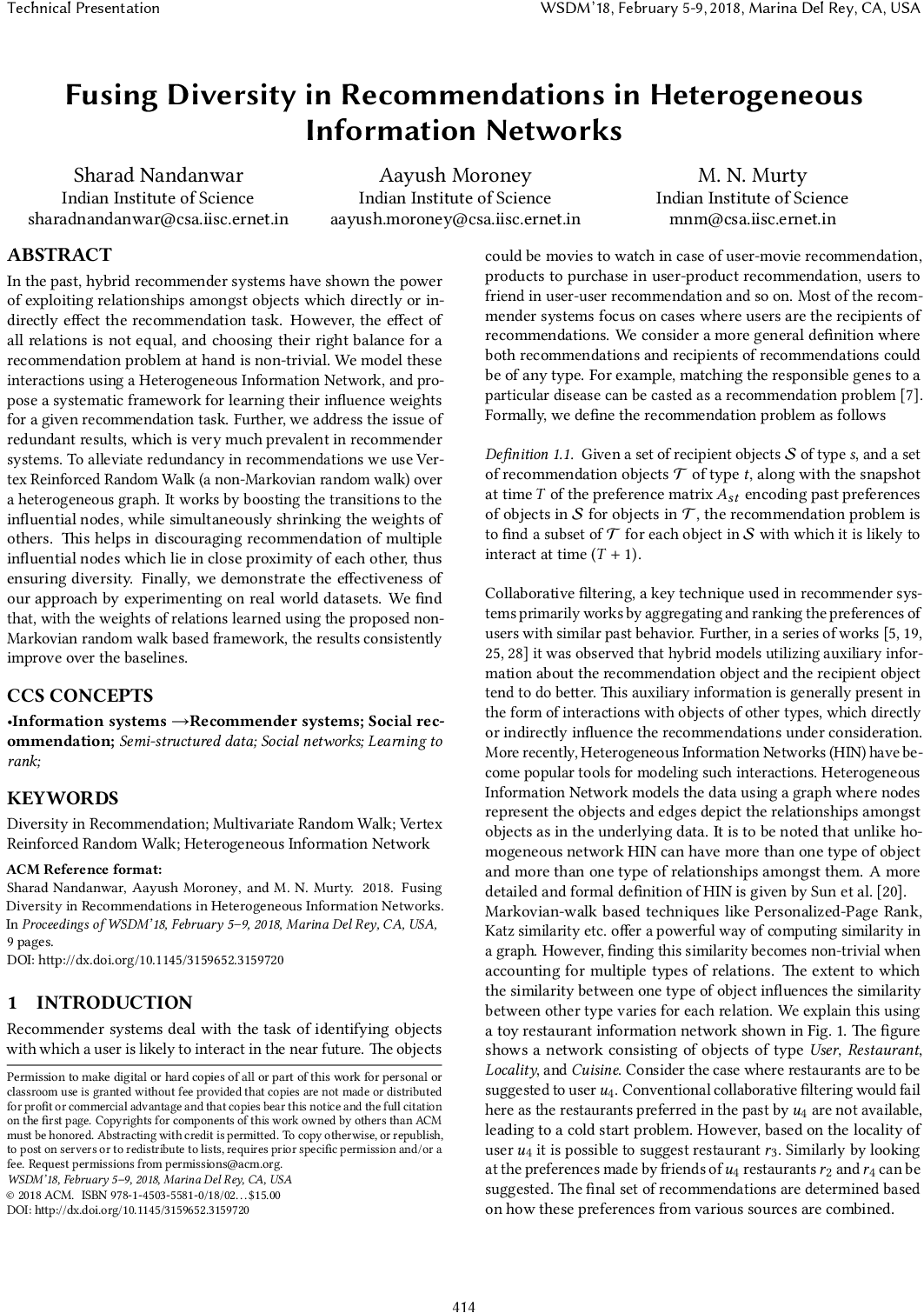
Authors:
Sharad Nandanwar,
Aayush Moroney,
M. N. Murty
Liked by:
Domains: Machine Learning, Recommendation
Tags: diversity, recommendation system, heterogeneous information networks
Liked by:
Domains: Machine Learning, Recommendation
Tags: diversity, recommendation system, heterogeneous information networks
Uploaded by:
Alt-Tab
Upload date: 2019-07-25 12:46:58
Upload date: 2019-07-25 12:46:58
Comments: